Может ли искусственный интеллект автоматизировать получение научных открытий?
Июль 2020
Опубликовано 2020-07-22 08:00
Опубликовано 2020-07-22 08:00
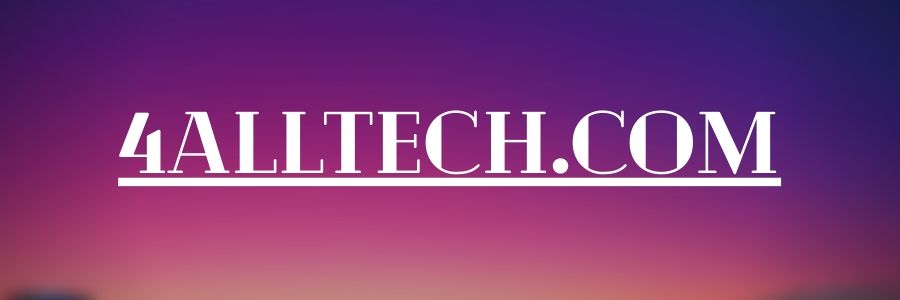
An AI reconstructs Newton's second law and discovers a previously unknown formula for mass calculation of dark matter. Can AI automate science?
AI in physics: are we facing a scientific revolution?
At the core of science are two essential components: observation and logic. The former generates data from which we can then use logic to identify regularities and formulate them in the language of mathematics.
Once formulated, the mathematical formula is more than just a description of the data - it enables us to make predictions and discover previously unknown relationships.
Why mathematics is so well suited to formulating natural laws is unclear. This fact is "a wonderful gift that we neither understand nor deserve," wrote the theoretical physicist and mathematician Eugene Wigner in his essay "The Unreasonable Effectiveness of Mathematics in the Natural Sciences".
Without the interaction of data and mathematics, our civilization would never have arisen. But the more extensive the data, the more complex the relationships, the longer it usually takes to find a mathematical formula that correctly describes the regularities in the data.
If you want to read a linear function from the data in a two-dimensional coordinate system in math lessons, you can do it in five minutes - or quickly watch a video on YouTube.The situation is different for more complex tasks: Physicists, for example, have been trying to combine quantum theory and relativity theory for almost a hundred years. And if this succeeds, it could take generations to clarify the effects, says physicist Lee Smolin .
If AI is like Columbus, computing power is Santa Maria
Can artificial intelligence accelerate the discovery of mathematical descriptions? If it's possible to automate science with computing power , scientific progress could be bound by Moore's law and increase so much.
AI models can already be used to model complex data relationships and make predictions. At its core, the process is simple: collect data, train AI, and make predictions. This works for a face detector as well as for an AI that can predict the movement of three bodies in space with high accuracy . AI learns and discovers relationships.
But the black box neural network makes it almost impossible to understand what is going on inside the network. And even if an insight were possible, the representation of the learned relationships in a deep neural network is far from the mathematical descriptions sought.
Symbolic regression produces mathematical description
The method of symbolic regression can change that. It can be used to derive mathematical formulas from the internally represented relationships in the network .
Why mathematics is so well suited to formulating natural laws is unclear. This fact is "a wonderful gift that we neither understand nor deserve," wrote the theoretical physicist and mathematician Eugene Wigner in his essay "The Unreasonable Effectiveness of Mathematics in the Natural Sciences".
Without the interaction of data and mathematics, our civilization would never have arisen. But the more extensive the data, the more complex the relationships, the longer it usually takes to find a mathematical formula that correctly describes the regularities in the data.
If you want to read a linear function from the data in a two-dimensional coordinate system in math lessons, you can do it in five minutes - or quickly watch a video on YouTube.The situation is different for more complex tasks: Physicists, for example, have been trying to combine quantum theory and relativity theory for almost a hundred years. And if this succeeds, it could take generations to clarify the effects, says physicist Lee Smolin .
If AI is like Columbus, computing power is Santa Maria
Can artificial intelligence accelerate the discovery of mathematical descriptions? If it's possible to automate science with computing power , scientific progress could be bound by Moore's law and increase so much.
AI models can already be used to model complex data relationships and make predictions. At its core, the process is simple: collect data, train AI, and make predictions. This works for a face detector as well as for an AI that can predict the movement of three bodies in space with high accuracy . AI learns and discovers relationships.
But the black box neural network makes it almost impossible to understand what is going on inside the network. And even if an insight were possible, the representation of the learned relationships in a deep neural network is far from the mathematical descriptions sought.
Symbolic regression produces mathematical description
The method of symbolic regression can change that. It can be used to derive mathematical formulas from the internally represented relationships in the network .
Symbolic regression is carried out as a genetic algorithm. Equipped with variables and mathematical operators, the algorithm searches for the simplest mathematical formula with which known data can be reproduced.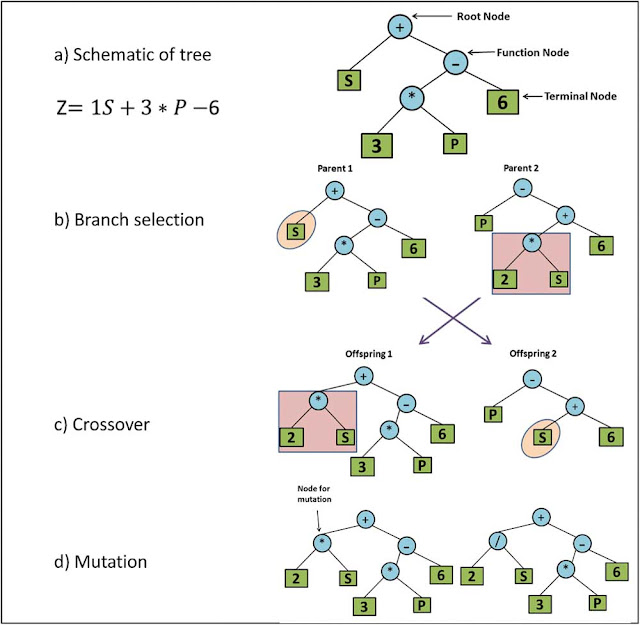
To do this, it generates a large number of formulas, compares their predictions with the known data and only adopts formulas that approximate the real data. The surviving formulas are then modified and measured again against each other. At the end of the process there is usually an approximately correct reproduction of the existing data and a simple mathematical formula.
From basic equations of mechanics
Researchers have now used this method to describe the movement of particles and the mass distribution of dark matter . For this they use so-called neural graph networks (GNN) .
These neural networks rely on graphs instead of layers arranged one after the other. Graphs consist of several vertices , which are connected to each other ( edges ). A node contains information that is passed on to the neighboring nodes via the connections and thus changes the state of the receiver point. In this way, each node gradually receives information about the entire graph.
Neural graph networks are particularly well suited for mapping physical systems, since these often consist of the interaction between individual units such as particles . If, for example, the movement of several particles is to be predicted, the nodes store information about the individual particles such as coordinates, last direction of movement and mass.
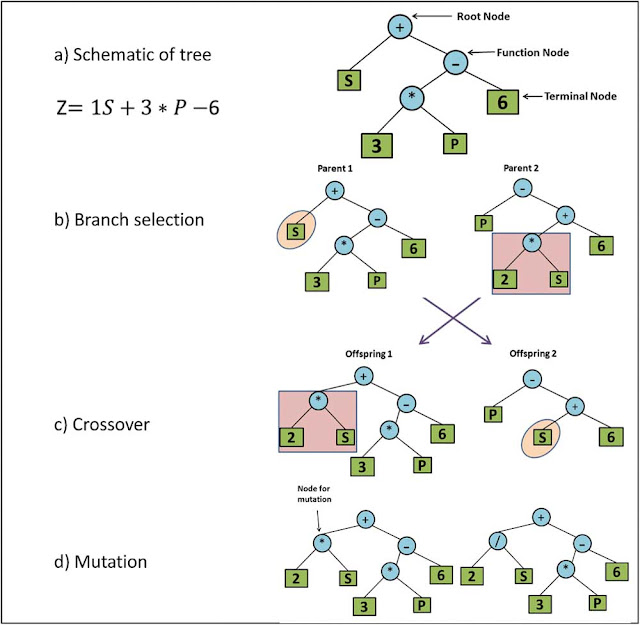
To do this, it generates a large number of formulas, compares their predictions with the known data and only adopts formulas that approximate the real data. The surviving formulas are then modified and measured again against each other. At the end of the process there is usually an approximately correct reproduction of the existing data and a simple mathematical formula.
From basic equations of mechanics
Researchers have now used this method to describe the movement of particles and the mass distribution of dark matter . For this they use so-called neural graph networks (GNN) .
These neural networks rely on graphs instead of layers arranged one after the other. Graphs consist of several vertices , which are connected to each other ( edges ). A node contains information that is passed on to the neighboring nodes via the connections and thus changes the state of the receiver point. In this way, each node gradually receives information about the entire graph.
Neural graph networks are particularly well suited for mapping physical systems, since these often consist of the interaction between individual units such as particles . If, for example, the movement of several particles is to be predicted, the nodes store information about the individual particles such as coordinates, last direction of movement and mass.
A pair of nodes corresponds to two interacting particles. The connections contain information about the forces acting on the respective particles (nodes) from the neighbors. The entire system can derive the acceleration of individual particles. If the GNN is trained with a data set of corresponding particle movements , its mapping of the interaction of the particles becomes better and better .
The researchers led by PhD student Miles Cranmer have now trained a GNN and have used symbolic regression to derive the basic equation of mechanics - force is mass times acceleration - from the network.
Their result clearly shows that the mixture of data, neural graph networks and symbolic regression is actually suitable for extracting mathematical formulas - in this case an already known natural law - from data with AI .
The researchers led by PhD student Miles Cranmer have now trained a GNN and have used symbolic regression to derive the basic equation of mechanics - force is mass times acceleration - from the network.
Their result clearly shows that the mixture of data, neural graph networks and symbolic regression is actually suitable for extracting mathematical formulas - in this case an already known natural law - from data with AI .
Calculate dark matter with AI
Spurred on by this success, Cranmer and colleagues turned to a current area of research in cosmology : they wanted to calculate a certain cosmological property (excessive density) of a dark matter accumulation depending on the properties of other dark matter accumulations in their environment.
Dark matter makes up about 85 percent of all matter in the universe, collects in huge structures and thus forms gravitational pools, so-called dark matter halos. The visible matter collects in these basins and forms stars and galaxies.
The researchers used the neural graphene network again. Each node contains information about a dark matter halo such as position, speed and mass and is connected to other halos at a distance of 50 Mpc / h. The network was trained with data from the Quijote Dark Matter Simulation , a collection of generated dark matter structures.
Spurred on by this success, Cranmer and colleagues turned to a current area of research in cosmology : they wanted to calculate a certain cosmological property (excessive density) of a dark matter accumulation depending on the properties of other dark matter accumulations in their environment.
Dark matter makes up about 85 percent of all matter in the universe, collects in huge structures and thus forms gravitational pools, so-called dark matter halos. The visible matter collects in these basins and forms stars and galaxies.
The researchers used the neural graphene network again. Each node contains information about a dark matter halo such as position, speed and mass and is connected to other halos at a distance of 50 Mpc / h. The network was trained with data from the Quijote Dark Matter Simulation , a collection of generated dark matter structures.
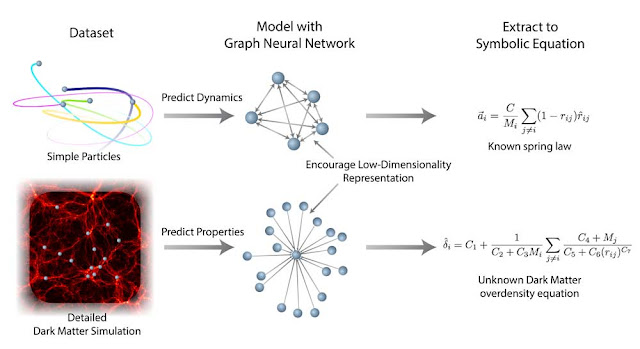
After the training, the GNN was able to predict the desired property of the halos more accurately than previous models. Using symbolic regression, the researchers were then able to produce a previously unknown mathematical formula that has a lower error rate than the currently most commonly used human-made formula for the same task.
The resulting formula was also better able to deal with previously unknown data. For Cranmer, this is a clear sign that the mathematical formula generalizes much better than the neural graph network from which it was derived. This coincides with our previous experience in physics, says Cranmer: "The language of simple symbolic models describes the universe correctly."
The success of Cranmer and his colleagues shows that the use of AI in research can lead to the discovery of previously unknown formulas . That would make the work of theoretical physicists easier. But the discovery itself is only the first step , after which the new formulas must be combined with known ones and derived. And we still need people to do that - for now.
Оставлять комментарии могут только зарегистрированные пользователи. Войдите в систему используя свою учетную запись на сайте: |
||